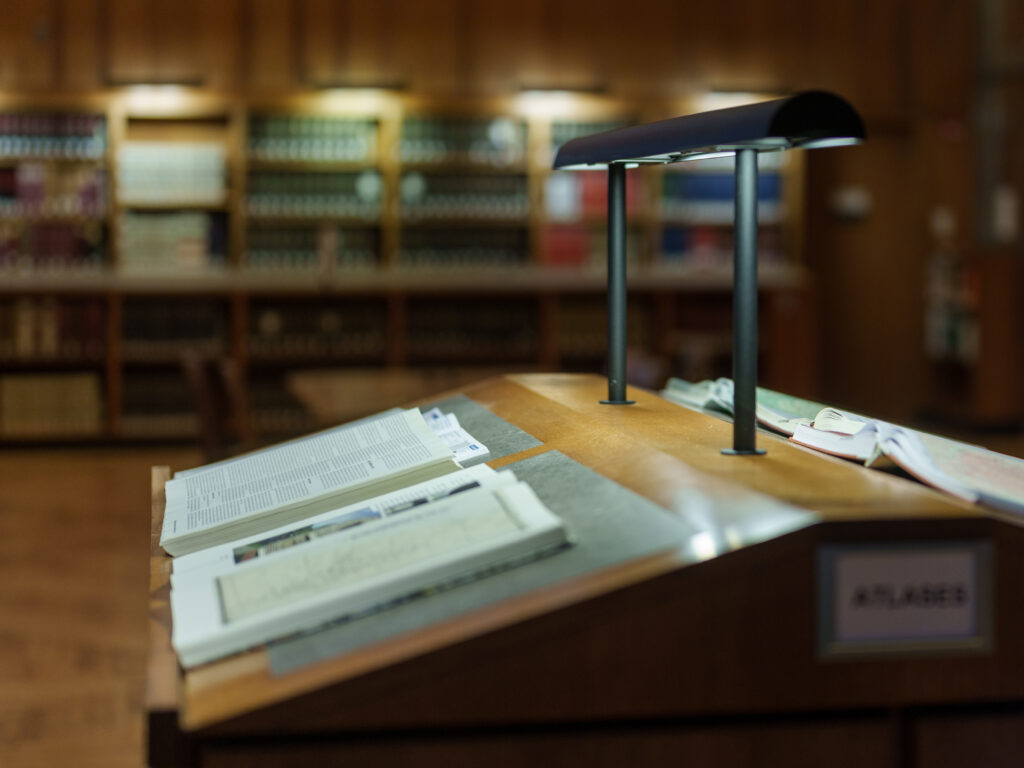
“So what does this data mean?” My professor asked, looking at me expectantly. What does the data mean? “What does this data tell you about the cancer cells?” If he thought rephrasing it made it any better, it didn’t. I am not quite sure what I said to save face (and frankly, I really do not want to remember), but I must have said something because my professor just nodded. “When you look at your data, I want you to create a story. It may be a mystery, but then you’d be providing a certain set of clues.”
It is very easy to get caught up in generating data, especially if the data is particularly tricky and you’re concerned about making sure it looks right, generating the right graphs, having the right axes, numbers and titles. It can be a headache. By the time the graphs are done, I would rather not look at it anymore or think too hard about the numbers. However, as lab reports and analysis questions stack up for our classes, it becomes prudent to know how to analyze these graphs. While I am not a seasoned veteran, I have a few tips that helped me approach these types of situations.
Continue reading Mystery Writers in Research Labs: How to Analyze Your Data